Share This: 
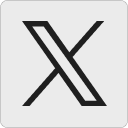

Examiner, Volume 8, Issue 3
October 2019
By Nicole Landi , Grace Ashton, Aviva Coyne-Green, Dan Kleinman, Heidi Sarles-Whittlesey, Pat Roberts, Nancy Blair, Kenneth Pugh, and Fumiko Hoeft
Educational neuroscience research on reading has been instrumental in building theoretical models of skilled and impaired reading (e.g., Gabrieli et al., 2016). Much of the work in this area is conducted in the lab using large equipment such as magnetic resonance imaging (MRI).
Despite notable advances in our understanding of the neural basis of reading and average treatment response, there remain missing links between brain imaging findings and practice (e.g., see Bowers, 2016 and associated commentaries). These gaps are also apparent when speaking with educators about how research influences their understanding of their students. Educators may wonder how to utilize results from studies examining children recruited for lab-based research involving researcher-guided treatments in their classrooms. These studies are well suited for identifying group differences (e.g., resisters vs. responders), but less well suited to identifying individual responses to in-situ reading intervention.
What about the students who fail to respond to the reading intervention that helps so many? Educators question why some students, who appear so similar at the beginning of the school year, improve at such different rates despite receiving the same intervention.
Beginning with our first in-school partnership between Haskins Laboratories and the AIM Institute for Learning and Research/AIM Academy, and now also with The Windward School, we hope to find these missing links and answer these questions.
Advances in Lab-Based Research
What have we learned about the neural basis of reading?
With neuroimaging techniques such as functional MRI (fMRI), several decades of research have identified a left hemisphere neural network for reading that builds off of regions that support spoken language processing and visual processing of complex stimuli (e.g. Pollack et al., 2015; Richlan et al., 2011). Interestingly, this neural circuitry for reading appears to be relatively consistent across languages despite differences in the opaqueness of grapheme to phoneme mappings (Martin et al., 2016; Rueckl et al., 2015).
This network is differentially activated (or used) during reading as a function of an individual’s skill level. Specifically, better readers typically show greater engagement of left hemisphere regions associated with recognizing visual word forms and accessing the phonological and semantic information associated with those forms (e.g., Hancock et al., 2017; Linkersdorfer et al., 2012; Richlan et al., 2009).
This additional activation is often interpreted as compensatory (see 2018 IDA Examiner article https://dyslexiaida.wpengine.com/compensatory-skills-and-dyslexia-what-does-the-science-say); that is, these regions are thought to come online to assist the less efficient “reading brain.” Moreover, hypoactivation and structural differences in left hemisphere regions are observed in high risk pre-readers suggesting that this “neural signature” might be causally related to poor reading (e.g., Martin et al., 2015; Richlan et al., 2013; Vandermosten et al., 2016).
…better readers typically show greater engagement of left hemisphere regions associated with recognizing visual word forms and accessing the phonological and semantic information associated with those forms.
Although much of the work in this area has been done using MRI, less expensive and more accessible methods (e.g., electroencephalography [EEG]) can also reliably index reading ability. Specifically, electrophysiological signatures (e.g., event related potential [ERP] components) of visual word recognition, phonological processing, and meaning access are all correlated with reading ability (e.g., Maurer et al., 2005; Hämäläinen et al., 2007; Landi & Perfetti, 2007, respectively). Some of these measures in pre-reading infants and toddlers are predictive of later reading skill, again suggesting that these neurobiological markers precede impairments in reading skill (Lohvansuu et al., 2018).
What have we learned about the neural basis of treatment response?
After mapping the neural circuitry for reading and identifying brain circuits that can discriminate typically from atypically developing readers, the logical next step in educational neuroscience was to determine how the brain changes in response to reading intervention—especially whether effective treatment moderates the brain differences seen in struggling and typically developing learners.
A number of these studies have found that children who make gains in reading show increased activation in left hemisphere (LH) reading circuits (e.g., Shaywitz et al., 2004) or reduction in atypical regions such as the right hemisphere (RH) homologues (i.e., counterparts) of these regions (Simos et al., 2002). Yet other studies claim that changes observed after intervention are primarily focused on the regions outside of the typical reading-related regions (Barquero et al., 2014; Xia et al., 2017). Although it is unclear whether these changes reflect “normalization” or a compensatory change, these findings do reveal a great deal of plasticity in the brain.
While this work reveals the neural response to intervention on average, it has now been established that not all children make these large gains in reading behavior. Indeed, initial estimates suggest that approximately 2-6% of children fail to make significant gains in reading even after substantial reading treatment (Torgesen, 2000), and these numbers likely underestimate the percentage of children with persistent difficulty in some domains.
One promising new direction for educational neuroscience is to identify neurobiological predictors and “biomarkers” of treatment response. If successful, these could give the field greater insight into why some learners respond to a given intervention, while others with similar reading challenges do not—ultimately making it possible to begin to use brain measures to better understand individual differences that impact treatment gains. Initial studies that looked at pre-to-post differences between treatment resisters and treatment responders found that responders were more likely to show the aforementioned left hemisphere increases (Shaywitz et al., 2004; Rezaie et al., 2011; Simos et al., 2007; Davis et al., 2011) and less likely to show right hemisphere increases (Simos et al., 2007). Other studies that focused on pre-treatment predictors found that initial right hemisphere activation and white matter structure (Hoeft et al., 2011), in addition to connections between frontal executive function networks and reading regions (Aboud et al., 2018), predicted successful treatment response.
While these initial efforts suggest that this is a fruitful line of inquiry, significant and important questions remain:
- Can we identify an individual student who is likely to be a resister before intervention?
- How different are patterns of pre-post neural change among students?
- Do distinct interventions change the brain differently?
- Can we identify the best intervention strategy based on these brain patterns?
With the work outlined in the next section, we hope to begin to answer some of these questions.
Haskins In-School Neuroscience Partnerships
The Haskins–school partnerships (with the AIM Academy and The Windward School) are part of a larger program of research that seeks to improve literacy globally. The goal of this program, the Haskins Global Language and Literacy (L2) Innovation Hub, is to improve language and literacy outcomes for children at risk for reading difficulties across languages and cultures.
This hub has four broad goals:
- Improving the sensitivity of early (birth-to-five) language assessment and intervention
- Delivering on the promise of neuroscience-guided reading instruction and/or remediation
- Scaling solutions to global challenges using education technology (ed-tech),
- Training a new generation of educators and clinicians (across cultures) to work with these emergent approaches.
Ongoing studies—in several countries (e.g., Mexico, Argentina, Peru, Brazil, and soon in India and China)—with children at risk for reading and educational outcome difficulties from poverty are using the neurocognitive approach at the heart of the hub to improve birth-to-eight language and literacy outcomes, and this work is expanding at the time of this writing.
The in-school laboratory research component of this larger program, which includes the Haskins-AIM Academy and Haskins-Windward School partnerships, is using EEG to identify neural signatures of treatment response in children with language-based learning difficulties undergoing treatment for poor reading, consistent with goal 2 above. By using this relatively accessible technology at frequent intervals as children progress through treatment, we hope to identify early indicators of which children will respond to standard evidence-based treatment and which children are more likely to have persistent problems. Further, we hope to identify why some of these children are failing to make adequate gains.
Although research from our labs and others identifies some promising correlates of persistent reading difficulty (e.g., processing speed, executive function) we have yet to identify causal relations between neurocognitive function and treatment response at the level of individual students. By identifying these causal factors and collaborating with those developing state-of-the-art interventions (including ed-tech), we hope to move away from a framework of resisters and responders toward a model of “what works for whom and why.”
Although research from our labs and others identifies some promising correlates of persistent reading difficulty (e.g., processing speed, executive function) we have yet to identify causal relations between neurocognitive function and treatment response at the level of individual students.
These Haskins-school partnerships extend beyond research. A key component of this program is a commitment to bidirectional training of students, teachers, clinicians, and practitioners (goal 4 above). This training links with the research arm, such that school staff members are critically involved in every step of the research, and likewise, researchers at all levels are integrating into the schools. This learning-by-doing model is complemented by formal training workshops that include research boot camps and lecture series.
Below is an outline of how the Haskins–AIM Academy partnership is making this possible:
- Preparation Phase 1: Once the research teams and schools found each other, there were numerous discussions on the vision, mission, and goals of the partnership. Strong leadership is critical when implementing a significant initiative or innovation in any field. This began at the board level, with a commitment to the importance of research on educational improvement and the understanding that all innovation requires financial and human capital investment. The AIM Board of Trustees provided leadership and mission-focused support that allowed all stakeholders—including faculty, parents, and the students themselves—to see the promise of this research-to-practice partnership. Without this broad community buy-in, the efforts of fund-raising and engagement in the research study itself would not have been possible. Where strong financial support is not available, foundation support may be a primary target through collaborations with existing programs such as this Haskins-AIM Academy initiative to show proof of concept and expert support to initiate a program.
- Preparation Phase 2: Once the infrastructure (e.g., funding and stake-holder buy-in) was in place, preparation for these projects involved substantial coordination of researchers and practitioners to agree upon common terms. This actually began far in advance as we started Preparation Phase 1 above. It involved a number of in-person meetings, within and across teams, to develop a research design and a data collection plan. After we agreed on a general plan for assessing students’ behavior and neural response at the beginning, middle, and end of the school year, we needed to establish space for data collection in the schools and to reserve time in students’ schedules. Identification of a time frame that would also provide meaningful data involved substantial coordination. In addition, we needed to hire research personnel, obtain approval from the universities and schools to conduct this research (IRB), obtain permission from parents and children, and order and install equipment for data collection.
- Execution: After completion of the preparation phase, we were ready to collect data. To date, we have installed one child-friendly EEG system for collection of behavioral and neurobiological data, and our equipment has arrived at two additional schools. We recently finished collection of Time 1 behavioral and neurobiological data from 30 children at AIM and Time 2 behavioral data in June. In addition, we have engaged nine educators and six high school students at AIM who have assisted with experimental procedures and EEG data collection. Presently, we are analyzing our data and preparing initial findings for dissemination.
- Next Steps: Moving forward, we are scaling up our data collection efforts to include more students in the schools. To maximize the contributions of individuals with non-research backgrounds, we are refining our data collection procedures to streamline our process and make it as user-friendly as possible. As part of this effort, we have hired additional staff and hosted several summer workshops to train more teachers and students in data collection. Our next step is to share our story more widely and involve additional school partners to ensure that our findings tell the most inclusive story of how diverse profiles of language learning difficulty are associated with different response profiles.
- Drivers for School Administrators: Today’s schools are facing a significant challenge in recruiting and retaining high quality educators. As AIM faculty have become more immersed in the research and implementation of the EEG assessments, as well as cutting-edge computer assessments, the level of inquiry and engagement has grown within the AIM professional community. This has created opportunities for faculty to participate in unique training workshops and simultaneously has created future career opportunities for the faculty. AIM has created two hybrid faculty positions for next school year, with salary enhancements, to provide release time for research participation and development of teacher training modules using AIM’s technology platform. Teacher passion and commitment fuel parent and student interest and add value to the overall educational experience offered. This is an educational eco-system that will engage the entire community in the all-important research-to-practice movement.
Teacher passion and commitment fuel parent and student interest and add value to the overall educational experience offered.
Future Directions
This work brings the neuroscience laboratory to the school and goes a long way toward forging some of the missing links outlined above by involving educational neuroscience researchers and educators in a common research process. The next test of our approach will come when we determine the reliability and predictive validity of our measures at the single-student level. We acknowledge that this will be an iterative process (i.e., repetition of a process in order to generate a sequence of outcomes) with multiple phases before we are able to meet our goal of identifying what works for whom and subsequently tailoring new interventions based on neurobiologically informed profiles.
As part of this iterative process, we have identified some longer-term future directions for our team:
- Addition of multimodal imaging techniques (i.e., multiple techniques that tap into different aspects of brain function and structure)
- Inclusion of brain-to-brain synchrony measures during online learning between students and between students and teachers.
EEG is small, portable, and may cost only thousands of dollars. It provides excellent information about nature and timing of sensory and cognitive processes. It is, however, fairly limited with respect to localization of function within the brain. Given that we know a lot about what specific regions in the reading network “do” and how their involvement changes as a function of reading intervention, the inclusion of measures that capture region-specific changes in activation will be valuable.
This work brings the neuroscience laboratory to the school and goes a long way toward forging some of the missing links outlined above by involving educational neuroscience researchers and educators in a common research process.
Although MRI can image the brain with exquisite spatial detail, it is expensive (millions of US dollars), large, and very vulnerable to movement contamination. An alternative is near-infrared spectroscopy (NIRS), with fridge-sized equipment, that costs a fraction of the expense of MRI (i.e., hundreds of thousands of dollars) and measures similar aspects of brain function as fMRI (though not in deep brain structures). It has the potential to provide information about region-specific activation changes without the downsides of MRI. Further, like EEG, NIRS systems can be installed in a school environment.
The second longer-term future direction involves capturing brain-to-brain synchrony measures that can be obtained using EEG or NIRS, in order to index online engagement—and, potentially, learning. Specifically, this approach involves simultaneous collection of neuroimaging data from two or more individuals and can reveal the neural basis of joint action or cognition. In a classroom setting, this approach can be used to measure neural synchrony between the teacher and the students or among the students. Thus far, this approach has revealed positive associations between student-teacher brain-to-brain synchrony and student engagement (Bevilacqua et al., 2019), and further use of this technique could explore the relation between neural synchrony and learning outcomes. The addition of these approaches would refine our neurobiological models of treatment response by linking regional (NIRS) and temporal (EEG) changes and by incorporating teacher-learner or intervention-learner interactions.
The vision of the Haskins Global L2 Innovation Hub and its partners begins with the goal of creating inter-connected community eco-systems that improve student outcomes through collaborative research, educational technology, and improved teacher practice in the classroom. This vision is already becoming a reality through internationally streamed research symposia, scalable student assessments, and high impact teacher training ed-tech solutions for researchers and educators across geographies, cultures, and languages—and it is impacting populations of children from diverse socio-economic backgrounds. This is the promise of linking research to practice to improve literacy for all children.
Learn More!
On November 7, at the upcoming IDA conference in Portland, Haskins will present more about its Haskins In-School Neuroscience Partnerships and EEG data collection to track children to identify individual treatment-response profiles. See the conference program brochure (Gordon F. Sherman Neuroscience and Education Symposium) for details.
References
Aboud, K. S., Barquero, L. A., & Cutting, L. E. (2018). Prefrontal mediation of the reading network predicts intervention response in dyslexia. Cortex, 101, 96–106. doi:10.1016/j.cortex.2018.01.009
Barquero, L. A., Davis, N., & Cutting, L. E. (2014). Neuroimaging of reading intervention: A systematic review and activation likelihood estimate meta-analysis. PloS one, 9(1), e83668. doi:10.1371/journal.pone.0083668
Bevilacqua, D., Davidesco, I., Wan, L., Chaloner, K., Rowland, J., Ding, M., … & Dikker, S. (2019). Brain-to-brain synchrony and learning outcomes vary by student–teacher dynamics: Evidence from a real-world classroom electroencephalography study. Journal of Cognitive Neuroscience, 31(3), 401-411. doi:10.1162/jocn_a_01274
Bowers, J. S. (2016). The practical and principled problems with educational neuroscience. Psychological Review, 123(5), 600. doi:10.1037/rev0000025
Davis, N., Barquero, L., Compton, D. L., Fuchs, L. S., Fuchs, D., Gore, J. C., & Anderson, A. W. (2011). Functional correlates of children’s responsiveness to intervention. Developmental Neuropsychology, 36(3), 288–301. doi:10.1080/87565641.2010.549875
Gabrieli, J. D. E. (2016). The promise of educational neuroscience: Comment on Bowers (2016). Psychological Review, 123(5), 613–619. doi:10.1037/rev0000034
Hämäläinen, J. A., Leppänen, P. H. T., Guttorm, T. K., & Lyytinen, H. (2007). N1 and P2 components of auditory event-related potentials in children with and without reading disabilities. Clinical Neurophysiology, 118(10), 2263–2275. doi:10.1016/j.clinph.2007.07.007
Hancock, R., Pugh, K. R., & Hoeft, F. (2017). Neural noise hypothesis of developmental dyslexia: Trends in Cognitive Sciences, 21(11), 909. doi:10.1016/j.tics.2017.08.003
Hoeft, F., McCandliss, B. D., Black, J. M., Gantman, A., Zakerani, N., Hulme, C., … & Gabrieli, J. D. (2011). Neural systems predicting long-term outcome in dyslexia. Proceedings of the National Academy of Sciences, 108(1), 361–366. doi:10.1073/pnas.1008950108
Landi, N., & Perfetti, C. A. (2007). An electrophysiological investigation of semantic and phonological processing in skilled and less skilled comprehenders. Brain and Language, 102(1), 30–45. doi:10.1016/j.bandl.2006.11.001
Linkersdörfer, J., Lonnemann, J., Lindberg, S., Hasselhorn, M., & Fiebach, C. J. (2012). Grey matter alterations co-localize with functional abnormalities in developmental dyslexia: an ALE meta-analysis. PloS one, 7(8), e43122. doi:10.1371/journal.pone.0043122
Lohvansuu, K., Hämäläinen, J. A., Ervast, L., Lyytinen, H., & Leppänen, P. H. (2018). Longitudinal interactions between brain and cognitive measures on reading development from 6 months to 14 years. Neuropsychologia, 108, 6–12. doi:10.1016/j.neuropsychologia.2017.11.018
Martin, A., Kronbichler, M., & Richlan, F. (2016). Dyslexic brain activation abnormalities in deep and shallow orthographies: A meta-analysis of 28 functional neuroimaging studies. Human Brain Mapping, 37(7), 2676–2699. doi:10.1002/hbm.23202
Martin, A., Schurz, M., Kronbichler, M., & Richlan, F. (2015). Reading in the brain of children and adults: a meta-analysis of 40 functional magnetic resonance imaging studies. Human Brain Mapping, 36(5), 1963–1981. doi:10.1002/hbm.22749
Maurer, U., Brem, S., Bucher, K., & Brandeis, D. (2005). Emerging neurophysiological specialization for letter strings. Journal of Cognitive Neuroscience, 17(10), 1532–1552. doi:10.1162/089892905774597218
Peck, F., Leong, A., Zekelman, L., & Hoeft, F. (2018). Compensatory skills and dyslexia: What does the science say? International Dyslexia Association, 7(2), Retrieved from https://dyslexiaida.wpengine.com/compensatory-skills-and-dyslexia-what-does-the-science-say/
Pollack, C., Luk, G., & Christodoulou, J. A. (2015). A meta-analysis of functional reading systems in typically developing and struggling readers across different alphabetic languages. Frontiers in Psychology, 6, 191. doi:10.3389/fpsyg.2015.00191
Rezaie, R., Simos, P. G., Fletcher, J. M., Cirino, P. T., Vaughn, S., & Papanicolaou, A. C. (2011). Temporo-parietal brain activity as a longitudinal predictor of response to educational interventions among middle school struggling readers. Journal of the International Neuropsychological Society, 17, 875–885. doi:10.1017/S1355617711000890
Richlan, F., Kronbichler, M., & Wimmer, H. (2009). Functional abnormalities in the dyslexic brain: a quantitative meta-analysis of neuroimaging studies. Human Brain Mapping, 30(10), 3299–3308. doi:10.1002/hbm.20752
Richlan, F., Kronbichler, M., & Wimmer, H., (2011). Meta-analyzing brain dysfunctions in dyslexic children and adults. NeuroImage, 56(3), 1735–1742. doi.10.1016/j.neuroimage.2011.02.040
Richlan, F., Kronbichler, M., & Wimmer, H., (2012). Structural abnormalities in the dyslexic brain: A meta-analysis of voxel-based morphometry studies. Human Brain Mapping, 34(11), 3055–3065. doi.10.1002/hbm.22127
Rueckl, J. G., Paz-Alonso, P. M., Molfese, P. J., Kuo, W. J., Bick, A., Frost, S. J., … & Lee, J. R. (2015). Universal brain signature of proficient reading: Evidence from four contrasting languages. Proceedings of the National Academy of Sciences, 112(50), 15510–15515. doi:10.1073/pnas.1509321112
Torgesen, J. K. (2000). Individual differences in response to early interventions in reading: The lingering problem of treatment resisters. Learning Disabilities Research & Practice, 15(1), 55–64.
Shaywitz, B. A., Shaywitz, S. E., Blachman, B. A., Pugh, K. R., Fulbright, R. K., Skudlarski, P., … & Fletcher, J. M. (2004). Development of left occipitotemporal systems for skilled reading in children after a phonologically-based intervention. Biological Psychiatry, 55(9), 926–933. doi:10.1016/j.biopsych.2003.12.019
Simos, P. G., Fletcher, J. M., Bergman, E., Breier, J. I., Foorman, B. R., Castillo, E. M., … & Papanicolaou, A. C. (2002). Dyslexia-specific brain activation profile becomes normal following successful remedial training. Neurology, 58(8), 1203–1213.
Simos, P. G., Fletcher, J. M., Sarkari, S., Billingsley-Marshall, R., Denton, C. A., & Papanicolaou, A. C. (2007). Intensive instruction affects brain magnetic activity associated with oral word reading in children with persistent reading disabilities. Journal of Learning disabilities, 40(1), 37–48. doi:10.1177/00222194070400010301
Vandermosten, M., Hoeft, F., & Norton, E. S. (2016). Integrating MRI brain imaging studies of pre-reading children with current theories of developmental dyslexia: A review and quantitative meta-analysis. Current Opinion in Behavioral Sciences, 10, 155–161. doi:10.1016/j.cobeha.2016.06.007
Xia, Z., Hancock, R., & Hoeft, F. (2017). Neurobiological bases of reading disorder Part I: Etiological investigations. Language and Linguistics Compass, 11(4), e12239. doi:10.1111/lnc3.12239
Copyright © 2019 International Dyslexia Association (IDA). Opinions expressed in The Examiner and/or via links do not necessarily reflect those of IDA.