Share This: 
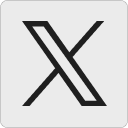

Volume 7, Issue 5
December 2018
By Richard K. Wagner, Florida State University and Florida Center for Reading Research
The research described in this article was supported by Grant Number P50 HD52120 and 1F31HD087054-01 from the National Institute of Child Health and Human Development.
Abstract Individuals with dyslexia are commonly misdiagnosed or even missed entirely. Part of the problem is unreliability in diagnosis that occurs for definitions that feature a single indicator, such as IQ-achievement discrepancy or RTI (Response to Intervention). A promising solution to this problem is the use of hybrid models that combine multiple indicators or criteria, thereby improving reliability of diagnosis. The constellation model that distinguishes causes, consequences, and correlates of dyslexia is one version of a hybrid model. When implemented as a Bayesian model, it incorporates both behavioral test data and biological factors (e.g., family history of reading problems) to estimate the probability that an individual has dyslexia. The model is flexible and can incorporate additional indicators (e.g., FMRI activation patterns or genetic markers) if and when they become available as predictors. Keywords: dyslexia, reading-disability, diagnosis, Bayesian models |
It is common for individuals with dyslexia to be misdiagnosed or even missed entirely.
The basic idea is to adapt important models that have become widely used in medicine to the problem of diagnosing dyslexia. For an example of such a model, consider the ASCVD calculator. If you are between the ages of 40 and 75, your next health checkup may provide 10-year and lifetime risk estimates for developing atherosclerotic cardiovascular disease (ASCVD)—likely to result in either death due to coronary heart disease, a nonfatal heart attack, or a stroke. The risk prediction will be made using a risk calculator (available online as http://tools.acc.org/ASCVD-Risk-Estimator/) that determines your risk based on your gender, age, race, HDL cholesterol, total cholesterol, systolic blood pressure, and whether you have a history of diabetes, smoking, or have been treated for hypertension. The ASCVD calculator is also designed to be used for estimating potential benefits from statin therapy by including it as a dependent variable in future statin therapy trials. An important and achievable goal is to build an analogous calculator that estimates risk of having dyslexia. In addition, the calculator could estimate probabilities relevant to functional outcomes, such as the probability that an individual would benefit from use of assistive technology (Wood, Moxley, Tighe, & Wagner, 2018) or the probability of responding to a particular intervention.
Why Is Diagnosis of Dyslexia Difficult?
It should be mentioned that diagnosis tends to be difficult regardless of the context. If you or someone you know has experienced a medical disease or a psychological or psychiatric disorder, more often than not, multiple diagnoses were made before arriving at a single correct one—if a correct diagnosis was ever achieved. Turning to dyslexia in particular, reading ability and disability represent ends of a continuous and likely normally distributed distribution. Figure 1 portrays this distribution with a gray shaded area representing the category of having dyslexia. The results of testing three individuals are displayed by lines with a small circle in the middle and bars at each end. The circle represents an individual’s true score or actual point on the continuum, and the line and bars represent the fact that measurement error causes an observed score to differ from a true score. This means that assessment results do not come out the same every time but vary randomly around an individual’s true score. For the individual on the left who is closest to the gray area, measurement error can cause the outcome of a diagnosis of dyslexia on one evaluation but not on another. This is less likely for individuals who are farther away from the cut-off.
Waesche, Schatschneider, Maner, Ahmed, and Wagner (2011) documented both poor agreement when alternative operational definitions of dyslexia were applied to the same sample and poor longitudinal stability of all the definitions. For example, when an aptitude-achievement discrepancy definition and an RTI-based definition were applied to the same sample, there was only 31% agreement. When an aptitude-achievement discrepancy definition and a low-achievement based definition were applied, there was only 32% agreement. One-year stabilities for diagnosis based on the alternative operational definitions of dyslexia were 24%, 34%, and 41% for the discrepancy, RTI, and low achievement operational definitions respectively. Others have reported similarly low levels of agreement and longitudinal stability (Barth et al., 2008; Fuchs, Fuchs, & Compton, 2004; Wagner, Waesche, Schatschneider, Maner, & Ahmed, 2011). The defining feature of these alternative operational definitions is that they prioritize a single indicator (e.g., poor decoding, inadequate response to instruction/intervention) over other factors. Consequently, these operational definitions are affected substantially by measurement error, and diagnosis becomes uncertain (Francis et al., 2005).
Approaches Toward More Accurate Diagnosis
A promising solution to this problem is the use of hybrid models that combine multiple indicators or criteria, thereby reducing the effects of measurement error (Wagner et al., 2011). A second promising solution is to avoid use of a cut-off score to decide whether dyslexia is present or absent—and, instead, to recognize the continuous nature of the criterion variable (i.e., different degrees of dyslexia ranging from none to mild to moderate to severe).
We refer to the hybrid model we have been investigating as a constellation model because it incorporates a constellation of symptoms and indicators instead of prioritizing a single indicator (Figure 2) (Wagner, Spencer, Quinn, & Tighe, 2013). The constellation model also differentiates causes, consequences, and correlates of dyslexia.
The model includes three causes:
- Impaired Phonological Processing. Regardless of the written script to be mastered, impaired phonological processing in general—and for alphabetic languages, impaired phonological awareness in particular—plays a causal role in the development of reading disability (Branum-Martin, Tao, Garnaat, Bunta, & Francis, 2012; Song, Georgiou, Su, & Hua, 2016; Swanson, Trainin, Necoechea, & Hammill, 2003).
- Genetic Risk. Genetic risk for reading disability has been shown by numerous behavioral genetic studies. Family history of reading disability, which can include both genetic and environmental components, increases the probability of having reading disability by a factor of four (Snowling & Melby-Lervag, 2016).
- Environmental Influences. Effective reading instruction and intervention appear to reduce the number of students who experience difficulty learning to read and may lessen the severity of reading disability for some students who remain impaired in reading (VanDerHeydan, Witt, & Gilbertson, 2007).
Family history of reading disability, which can include both genetic and environmental components, increases the probability of having reading disability by a factor of four (Snowling & Melby-Lervag, 2016).
Turning to consequences, which also serve as indicators of the latent construct of reading disability in our model, four proximal consequences are included:
- Poor Decoding (e.g., accuracy and fluency of nonword decoding) (Hermann, Matyas, & Pratt, 2006; Lyon, Shaywitz, & Shaywitz, 2003; Stanovich, 1988)
- Impoverished Sight-Word Vocabulary (e.g., automaticity of real word decoding) (Ehri, 1988)
- Poor Response to Instruction and Intervention (Fletcher & Vaughn, 2009)
- Listening Comprehension That Is Better Than Reading Comprehension (Badian, 1999; Stanovich, 1991; Spencer et al., 2014)
In a large-scale study (N = 31,339) of students who were followed longitudinally from 1st to 2nd grade, Spencer et al. (2014) implemented two versions of a model of reading disability based on the four consequences listed above. The first version, which was dimensional, modeled reading disability as a latent variable with the four consequences as observed indicators. Confirmatory factor analysis yielded an excellent model fit ([χ2(2) = 263.4; Comparative Fit Index = .99; Tucker Lewis Index = .97; Root Mean Square Error of Approximation = .065 with a 95% confidence interval between .058 and .071; note that the large chi-square is expected because of the sample size). The one-year stability of this dimensional version of the model was substantial with a standardized structure coefficient of 0.88. This means that over 75% of the variance in the latent variable representation of reading disability in 2nd grade was accounted for by 1st grade performance. In order to better compare it to existing alternative categorical models, the second model was categorical rather than dimensional. The implementation was based on the number of indicators that were present instead of their continuous levels as in the first version of the model. The one-year stability of various versions of this model exceeded that of traditional, single-indicator models, with the best performing versions of the model approaching twice the stability of single-indicator traditional models.
Referring back to the ASCVD risk calculator, the underlying model used to estimate risk is based on a Bayesian regression model that used biographical information (e.g., gender, age, and race), historical information (e.g., history of diabetes, smoking, and treatment for hypertension), and recently collected data (HDL cholesterol, total cholesterol, systolic blood pressure) to predict heart attack and stroke. Bayesian models are flexible models that can incorporate multiple kinds of information. They are particularly useful when informative priors are available. In the present context, informative priors are baseline probabilities that represent the prevalence of something in the population in general. This population prevalence or prior probability is then updated based on information for particular individuals.
Bayesian models are commonly used in medical diagnosis and policy (Spiegelhalter, Abrams, & Myles, 2004). For example, it has been recommended that women in their 40s do not get routine mammograms unless they have a family history of breast cancer or other known risk factors. The reason is that breast cancer is rare for women in their 40s, which translates into a low prior probability. Mammography is not completely accurate; following up a positive mammogram when breast cancer is not present carries a risk of complications. A woman in her 40s with no known risk factors who gets a positive mammogram still has only around a 10% chance of actually having breast cancer.
For an example of using a Bayes model to estimate the probability of the presence of dyslexia, we operationally defined dyslexia as scoring at or below the 5th percentile on a factor score for the factor depicted in Figure 3. Because we chose the 5th percentile, the prior probability or chance of having dyslexia is 5%. We can then update this probability on the basis of whether an individual is male or female, because boys are about two times more likely to have severe cases of dyslexia than girls. If the individual is female, the chance of having dyslexia decreases from 5% to 3%; if male, the chance increases from 5% to 7%. Scoring at or below the 20th percentile on a battery of 1st-grade predictors triples the chances of having dyslexia, from 5% to 15%. Co-occurring ADHD increases the chances of having dyslexia fourfold, from 5% to 19%. Having an affected parent or sibling increases the chances fivefold, from 5% to 26%. Combinations of risk factors have an even greater effect on risk. For example, being male with ADHD increases the chance from 5% to 24%. Add in an affected parent or sibling, and the chances increase to 76%. Finally, add a low score on the predictor battery, and probability increases to 92%!
In summary, existing definitions of dyslexia do not result in reliable diagnosis because they rely primarily on a single indicator. Expanding definitions to include multiple indicators improves the reliability of diagnosis.
In summary, existing definitions of dyslexia do not result in reliable diagnosis because they rely primarily on a single indicator. Expanding definitions to include multiple indicators improves the reliability of diagnosis. Moving to Bayesian models with informative priors should result in additional improvement in diagnosis.
References
Badian, N. A. (1999). Reading disability defined as a discrepancy between listening and reading comprehension: A longitudinal study of stability, gender differences, and prevalence. Journal of Learning Disabilities, 32(2), 138–148. doi:1177/002221949903200204
Barth, A. E., Stuebing, K. K., Anthony, J. L., Denton, C. A., Mathes, P. G., Fletcher, J. M., & Francis, D. J. (2008). Agreement among response to intervention criteria for identifying responder status. Learning and Individual Differences, 18, 296–307. doi:10.1016/j.lindif.2008.04.004
Branum-Martin, L., Tao, S., Garnaat, S., Bunta, F., & Francis, D. J. (2012). Meta-analysis of bilingual phonological awareness: Language, age, and psycholinguistic grain size. Journal of Educational Psychology, 104(4), 932–944. doi:10.1037/a0027755
Ehri, L. C. (1988). Grapheme-phoneme knowledge is essential for learning to read words in English. In J. L. Metsala & L. C. Ehri (Eds.), Learning and teaching reading. London: British Journal of Educational Psychology Monograph Series II.
Fletcher, J. M., & Vaughn, S. (2009). Response to intervention: Preventing and remediating academic difficulties. Child Development Perspectives, 3, 30–37. doi:10.1111/j.1750-8606.2008.00072.x
Francis, D. J., Fletcher, J. M., Stuebing, K. K., Lyon, G. R., Shaywitz, B. A., & Shaywitz, S. E. (2005). Psychometric approaches to the identification of LD: IQ and achievement scores are not sufficient. Journal of Learning Disabilities, 38, 98–108. doi:10.1177/00222194050380020101
Fuchs, D., Fuchs, L. S., & Compton, D. (2004). Identifying reading disabilities by responsiveness-to-instruction: Specifying measures and criteria. Learning Disability Quarterly, 27, 216–227. doi:10.2307/1593674
Herrmann, J. A., Matyas, T., & Pratt, C. (2006). Meta-analysis of the nonword reading deficit in specific reading disorder. Dyslexia: An International Journal of Research and Practice, 12(3), 195–221. doi:10.1002/dys.324
Lyon, G. R., Shaywitz, S. E., & Shaywitz, B. A. (2003). A definition of dyslexia. Annals of Dyslexia, 53, 1–14. doi:10.1007/s11881-003-0001-9
Quinn, J. M., & Wagner, R. K. (2015). Gender differences in reading impairment and the identification of impaired readers: Results from a large-scale study of at-risk readers. Journal of Learning Disabilities, 48(4), 433–445. doi:10.1177/0022219413508323
Snowling, M. J., & Melby-Lervåg, M. (2016). Oral language deficits in familial dyslexia: A meta-analysis and review. Psychological Bulletin, 142(5), 498–545. doi:10.1037/bul0000037
Song, S., Georgiou, G. K., Su, M., & Hua, S. (2016). How well do phonological awareness and rapid automatized naming correlate with Chinese reading accuracy and fluency? A meta-analysis. Scientific Studies of Reading, 20(2), 99–123. doi:10.1080/10888438.2015.1088543
Spencer, M., Wagner, R. K., Schatschneider, C., Quinn, J. M., Lopez, D., & Petscher, Y. (2014). Incorporating RTI in a hybrid model of reading disability. Learning Disability Quarterly, 37, 161–171. doi:10.1177/0731948714530967
Spiegelhalter, D. J., Abrams, K. R., & Myles, J. P. (2004). Bayesian approaches to clinical trials and health-care evaluation. West Sussex, England: John Wiley & Sons.
Stanovich, K. E. (1988). Explaining the differences between the dyslexic and the garden-variety poor reader: The phonological-core variable-difference model. Journal of Learning Disabilities, 21, 590–604. doi:10.1177/002221948802101003
Stanovich, K. E. (1991). Conceptual and empirical problems with discrepancy definitions of reading disability. Learning Disability Quarterly, 14(4), 269–280. doi:10.2307/1510663
Swanson, H. L., Trainin, G., Necoechea, D. M., & Hammill, D. D. (2003). Rapid naming, phonological awareness, and reading: A meta-analysis of the correlation evidence. Review of Educational Research, 73(4), 407–440. doi:10.3102/00346543073004407
VanDerHeyden, A. M., Witt, J. C., & Gilbertson, D. (2007). A multi-year evaluation of the effects of a response to intervention (RTI) model on identification of children for special education. Journal of School Psychology, 45(2), 225–256. doi:10.1016/j.jsp.2006.11.004
Waesche, J. B., Schatschneider, C., Maner, J. K., Ahmed, Y., & Wagner, R. K. (2011). Examining agreement and longitudinal stability among traditional and RTI-based definitions of reading disability using the affected-status agreement statistic. Journal of Learning Disabilities, 44, 296–307. doi:10.1177/0022219410392048
Wagner, R. K., Spencer, M., Quinn, J. M., & Tighe, E. L. (2013, November). Towards a more stable phenotype of reading disability. Paper presented at the 8th Biennial Meeting of the Society for the Study of Human Development (SSHD), Fort Lauderdale, FL, USA.
Wagner, R. K., Waesche, J. B., Schatschneider, C., Maner, J. K., & Ahmed, Y. (2011). Using response to intervention for identification and classification. In P. McCardle, J. R. Lee, B. Miller, & O. Tzeng (Eds.), Dyslexia across languages: Orthography and the brain-gene-behavior link (pp. 202–213). Baltimore, MD: Brookes Publishing.
Willcutt, E. G., DeFries, J. C., Pennington, B. F., Smith, S. D., Cardon, L. R., & Olson, R. K. (2003). Genetic etiology of comorbid reading difficulties and ADHD. In R. Plomin, J. C. DeFries, I. W. Craig, & P. McGuffin (Eds.), Behavioral genetics in the postgenomic era (pp. 227–246). Washington, DC: American Psychological Association. doi:10.1037/10480-013
Wood, S. G., Moxley, J. H., Tighe, E. L., & Wagner, R. K. (2018). Does use of text-to-speech and related read-aloud tools improve reading comprehension for students with reading disabilities? A meta-analysis. Journal of Learning Disabilities, 51, 73–84. doi:10.1177/0022219416688170
Richard K. Wagner, PhD, Robert O. Lawton Distinguished Research Professor of Psychology and the W. Russell and Eugenia Morcom Chair at Florida State University, has focused his research on dyslexia and the normal acquisition of reading. He helped to coin the term phonological processing with his paper “The Nature of Phonological Processing and Its Causal Relations with Reading” to describe related work on phonological awareness, phonological memory, and rapid naming that had previously been viewed as three separate domains of research. Most notably, dismayed by the lack of knowledge and tools available to school psychologists and professionals to help with evaluation of children with dyslexia and other learning differences, Dr. Wagner worked to develop the Comprehensive Test of Phonological Processing (CTOPP-2), the Test of Word Reading Efficiency (TOWRE-2), and the Test of Preschool Early Literacy (TOPEL).
Copyright © 2018 International Dyslexia Association (IDA). Opinions expressed in The Examiner and/or via links do not necessarily reflect those of IDA.
We encourage sharing of Examiner articles. If portions are cited, please make appropriate reference. Articles may not be reprinted for the purpose of resale. Permission to republish this article is available from info@dyslexia.org.